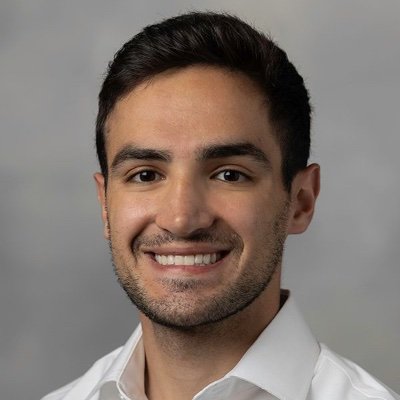
Cyril Zakka, MD
Hi! I'm a medical doctor, iOS/macOS developer, and ML researcher.
My research interests primarily involve building, training, and evaluating multimodal large language models (MLLM) for clinical medicine, as well as foundation models for surgery and cardiac imaging.
Timeline
Hugging Face Moonshot
ML Researcher, Health AI LeadDepartment of Cardiothoracic Surgery, Stanford Medicine
Postdoctoral FellowshipAmerican University of Beirut Medical Center
Medical Doctorate DegreeBoston College
Bachelor of Science in Biology
Blog Posts
Research
Radiology
Best Practices for Large Language Models in Radiology
Radiologists must integrate complex imaging data with clinical information to produce actionable insights. This task requires a nuanced application of language across many activities, including managing clinical requests, analyzing imaging findings in the context of clinical data, interpreting these through the radiologist’s lens, and effectively documenting and communicating the outcomes. Radiology practices must ensure reliable communication among numerous systems and stakeholders critical for medical decision-making. Large language models (LLMs) offer an opportunity to improve the management and interpretation of the vast amounts of text data in radiology. Despite being developed as general-purpose tools, these advanced computational models demonstrate impressive capabilities in specialized tasks, even without specific training. Unlocking the potential of LLMs for radiology requires an understanding of their foundations and a strategic approach to navigate their idiosyncrasies. This review, drawing from practical radiology and machine learning expertise, provides general and technically adept radiologists insight into the potential of LLMs in radiology. It also equips those interested in implementing applicable best practices that have so far stood the test of time in the rapidly evolving landscape of LLMs. The review provides practical advice for optimizing LLM characteristics for radiology practices, including advice on limitations, effective prompting, and fine-tuning strategies.
arXiv
SmolLM2: When Smol Goes Big--Data-Centric Training of a Small Language Model
While large language models have facilitated breakthroughs in many applications of artificial intelligence, their inherent largeness makes them computationally expensive and challenging to deploy in resource-constrained settings. In this paper, we document the development of SmolLM2, a state-of-the-art "small" (1.7 billion parameter) language model (LM). To attain strong performance, we overtrain SmolLM2 on ~11 trillion tokens of data using a multi-stage training process that mixes web text with specialized math, code, and instruction-following data. We additionally introduce new specialized datasets (FineMath, Stack-Edu, and SmolTalk) at stages where we found existing datasets to be problematically small or low-quality. To inform our design decisions, we perform both small-scale ablations as well as a manual refinement process that updates the dataset mixing rates at each stage based on the performance at the previous stage. Ultimately, we demonstrate that SmolLM2 outperforms other recent small LMs including Qwen2.5-1.5B and Llama3.2-1B. To facilitate future research on LM development as well as applications of small LMs, we release both SmolLM2 as well as all of the datasets we prepared in the course of this project.
arXiv
MediSyn: Text-Guided Diffusion Models for Broad Medical 2D and 3D Image Synthesis
Diffusion models have recently gained significant traction due to their ability to generate high-fidelity and diverse images and videos conditioned on text prompts. In medicine, this application promises to address the critical challenge of data scarcity, a consequence of barriers in data sharing, stringent patient privacy regulations, and disparities in patient population and demographics. By generating realistic and varying medical 2D and 3D images, these models offer a rich, privacy-respecting resource for algorithmic training and research. To this end, we introduce MediSyn, a pair of instruction-tuned text-guided latent diffusion models with the ability to generate high-fidelity and diverse medical 2D and 3D images across specialties and modalities. Through established metrics, we show significant improvement in broad medical image and video synthesis guided by text prompts.
arXiv
Almanac Copilot: Towards Autonomous Electronic Health Record Navigation
Clinicians spend large amounts of time on clinical documentation, and inefficiencies impact quality of care and increase clinician burnout. Despite the promise of electronic medical records (EMR), the transition from paper-based records has been negatively associated with clinician wellness, in part due to poor user experience, increased burden of documentation, and alert fatigue. In this study, we present Almanac Copilot, an autonomous agent capable of assisting clinicians with EMR-specific tasks such as information retrieval and order placement. On EHR-QA, a synthetic evaluation dataset of 300 common EHR queries based on real patient data, Almanac Copilot obtains a successful task completion rate of 74% (n = 221 tasks) with a mean score of 2.45 over 3 (95% CI:2.34-2.56). By automating routine tasks and streamlining the documentation process, our findings highlight the significant potential of autonomous agents to mitigate the cognitive load imposed on clinicians by current EMR systems.
JAMA Cardiology
The STOP-RVF Score: Machine Learning Multicenter Risk Model to Predict Right Ventricular Failure After Mechanical Circulatory Support
The existing models predicting right ventricular failure (RVF) after durable left ventricular assist device (LVAD) support might be limited, partly due to lack of external validation, marginal predictive power, and absence of intraoperative characteristics. The objective of this study is to derive and validate a risk model to predict RVF after LVAD implantation. This was a hybrid prospective-retrospective multicenter cohort study conducted from April 2008 to July 2019 of patients with advanced heart failure (HF) requiring continuous-flow LVAD. The derivation cohort included patients enrolled at 5 institutions. The external validation cohort included patients enrolled at a sixth institution within the same period. Study data were analyzed October 2022 to August 2023. The primary outcome was RVF incidence, defined as the need for RV assist device or intravenous inotropes for greater than 14 days. Bootstrap imputation and adaptive least absolute shrinkage and selection operator variable selection techniques were used to derive a predictive model. An RVF risk calculator (STOP-RVF) was then developed and subsequently externally validated, which can provide personalized quantification of the risk for LVAD candidates. Its predictive accuracy was compared with previously published RVF scores.
NEJM-AI
Almanac: Retrieval-Augmented Language Models for Clinical Medicine
Large language models (LLMs) have recently shown impressive zero-shot capabilities, whereby they can use auxiliary data, without the availability of task-specific training examples, to complete a variety of natural language tasks, such as summarization, dialogue generation, and question answering. However, despite many promising applications of LLMs in clinical medicine, adoption of these models has been limited by their tendency to generate incorrect and sometimes even harmful statements. We tasked a panel of eight board-certified clinicians and two health care practitioners with evaluating Almanac, an LLM framework augmented with retrieval capabilities from curated medical resources for medical guideline and treatment recommendations. The panel compared responses from Almanac and standard LLMs (ChatGPT-4, Bing, and Bard) versus a novel data set of 314 clinical questions spanning nine medical specialties. Almanac showed a significant improvement in performance compared with the standard LLMs across axes of factuality, completeness, user preference, and adversarial safety.
arXiv
A Generalizable Deep Learning System for Cardiac MRI
Cardiac MRI allows for a comprehensive assessment of myocardial structure, function, and tissue characteristics. Here we describe a foundational vision system for cardiac MRI, capable of representing the breadth of human cardiovascular disease and health. Our deep learning model is trained via self-supervised contrastive learning, by which visual concepts in cine-sequence cardiac MRI scans are learned from the raw text of the accompanying radiology reports. We train and evaluate our model on data from four large academic clinical institutions in the United States. We additionally showcase the performance of our models on the UK BioBank, and two additional publicly available external datasets. We explore emergent zero-shot capabilities of our system, and demonstrate remarkable performance across a range of tasks; including the problem of left ventricular ejection fraction regression, and the diagnosis of 35 different conditions such as cardiac amyloidosis and hypertrophic cardiomyopathy. We show that our deep learning system is capable of not only understanding the staggering complexity of human cardiovascular disease, but can be directed towards clinical problems of interest yielding impressive, clinical grade diagnostic accuracy with a fraction of the training data typically required for such tasks.
M4LH
Med-Flamingo: A Multimodal Few Shot Learner
Medicine, by its nature, is a multifaceted domain that requires the synthesis of information across various modalities. Medical generative vision-language models (VLMs) make a first step in this direction and promise many exciting clinical applications. We propose Med-Flamingo, a multimodal few-shot learner adapted to the medical domain. Based on OpenFlamingo-9B, we continue pre-training on paired and interleaved medical image-text data from publications and textbooks. Med-Flamingo unlocks few-shot generative medical visual question answering (VQA) abilities, which we evaluate on several datasets including a novel challenging open-ended VQA dataset of visual USMLE-style problems.